business resources
AI Readiness Scorecard To Evaluate Organisational Preparedness For AI Transformation
22 Apr 2025, 6:19 am GMT+1
AI Readiness Scorecard To Evaluate Organisational Preparedness For AI Transformation
The AI Readiness Scorecard offers a framework for organisations to assess their preparedness for integrating artificial intelligence (AI). How ready is your business (or organisation) making an impact to bridge the gap between information, knowledge, and the wisdom that is necessary to effectively harness AI. The AI Readiness Scorecard is both a mirror and a map, a reflection of current capabilities while being a guide for businesses and organisations to the way forward.
AI adoption goes beyond technological change; it marks a profound transformation in how organisations function, compete, and create value.
As noted by Yuval Noah Harari in Homo Deus, "Once technology enables us to re-engineer human minds, Homo sapiens will disappear, human history will come to an end, and a completely new process will begin."
While not as dramatic in a business context, AI adoption signals a profound transformation.
The imperative of AI readiness for businesses
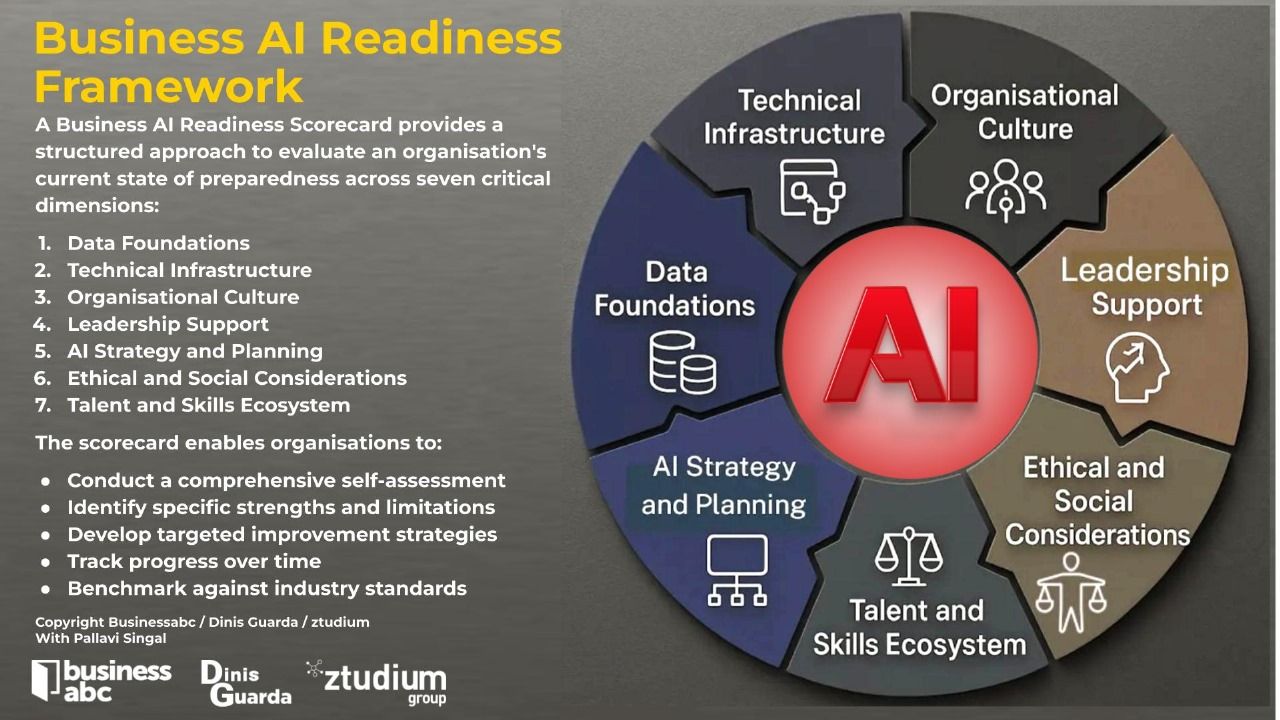
In today’s rapidly evolving business landscape, AI adoption is no longer optional—it’s essential for remaining competitive. The AI Readiness Scorecard provides a structured framework for organisations to assess their preparedness across seven critical dimensions:
- Data Foundations: Data is the lifeblood of AI. Without robust, clean, and structured data, AI systems cannot function effectively. Businesses like Netflix and Amazon leverage vast amounts of customer data to personalise recommendations and improve user experience. For Example: Companies such as Siemens have invested heavily in creating comprehensive data lakes and ensuring data cleanliness before deploying AI models.
- Technical Infrastructure: AI requires advanced technical infrastructure, including powerful computing resources, storage systems, and cloud solutions. Companies like Tesla and Google have built state-of-the-art infrastructure to support their AI-driven innovations, from self-driving cars to AI research in DeepMind. Use Case: Microsoft’s Azure AI platform offers organisations scalable infrastructure to run machine learning models, ensuring businesses have the resources they need to process and analyse vast data sets in real-time.
- Organisational Culture: AI adoption thrives in a culture that embraces innovation, agility, and continuous learning. Example: Spotify has integrated AI-driven recommendation systems that allow employees to experiment and learn, fostering a culture of innovation where data-driven decisions are encouraged across departments.
- Leadership Support: Strong leadership is key to championing AI initiatives and ensuring alignment with business objectives. At companies like Google, AI leadership comes from the top down, with executives like Sundar Pichai setting clear AI-focused goals and investing in research and development. Use Case: Accenture’s CEO, Julie Sweet, has been vocal about AI’s role in transforming business processes, pushing for AI integration into consulting services to better serve clients.
- AI Strategy and Planning: Without a clear AI strategy, businesses risk wasting time and resources. An AI strategy should include goals such as improving operational efficiency, enhancing customer experience, or developing new products. Real-World Example: Uber uses AI to predict ride demand and optimize driver assignments. Their AI strategy focuses on customer satisfaction, fleet management, and cost reduction, with clear KPIs to track progress.
- Ethical and Social Considerations: As AI becomes more integrated into business operations, organisations must address ethical concerns, including bias, fairness, privacy, and accountability. Example: IBM has led the way in developing AI ethics frameworks, particularly through their AI Fairness 360 toolkit, which helps organisations reduce bias in AI models.
- Talent and Skills Ecosystem: AI success hinges on having the right talent with expertise in data science, machine learning, and AI ethics. Use Case: Google DeepMind hires top-tier scientists, data engineers, and ethicists to push the boundaries of AI research while addressing complex ethical issues related to AI deployment.
How the AI Readiness Scorecard Helps Organisations:
- Conduct a Self-Assessment: Companies can use the scorecard to evaluate their current position in each of the seven dimensions, gaining insights into where they stand in their AI journey.
- Identify Strengths and Limitations: By highlighting areas of strength and weaknesses, businesses can determine which elements require further investment, whether in data infrastructure or ethical AI training.
- Develop Targeted Improvement Strategies: Based on the assessment, businesses can create targeted strategies to strengthen weak areas. For instance, a retail business might focus on building an AI strategy and culture to better understand consumer behaviour and improve inventory management.
- Track Progress: The scorecard helps businesses track progress over time, making it easier to measure AI adoption and its business impact.
- Benchmark Against Industry Standards: By comparing their AI readiness with industry standards, businesses can set realistic goals and better align with market trends.
Methodology
The AI Readiness Scorecard uses both quantitative and qualitative assessments. Each dimension contains several evaluation criteria, rated on a 5-point maturity scale:
- Nascent (1 point): Limited or no capabilities
- Emerging (2 points): Basic foundations with gaps
- Developing (3 points): Moderate capabilities with ongoing implementation
- Advanced (4 points): Strong capabilities with some refinement needed
- Leading (5 points): Exceptional capabilities representing best practices
Dimension 1: Data Foundations
"Data is the new oil. It's valuable, but if unrefined it cannot really be used." — Clive Humby
The evaluation criteria include data quality and accessibility, governance, integration, volume and variety, and privacy and security.
An organisation's data maturity is crucial for AI success, with the scorecard assessing data quality, integration, and privacy measures across a 1-5 scale. A sophisticated data governance structure, high-quality data standards, and seamless integration are key elements for organisations striving to lead in AI.
Dimension 2: Technical Infrastructure
Organisations must have the technical infrastructure to support AI workloads. This dimension evaluates computing resources, AI development environments, API and integration capabilities, technology stack maturity, and cloud scalability. As AI demands specialised computing resources, organisations must ensure they possess the necessary infrastructure to manage and scale AI initiatives effectively.
A state-of-the-art infrastructure optimised for AI workloads is vital for organisations seeking to become leaders in AI transformation. Cloud adoption and scalability are also important factors in evaluating the technical infrastructure.
Dimension 3: Organisational Culture
AI adoption is not just a technical change but also a cultural one. An innovation-driven mindset, data-driven decision making, cross-functional collaboration, change readiness, and continuous learning are all essential components of this dimension.
For organisations to succeed in their AI journey, they need a culture that embraces innovation and change. This includes fostering a collaborative environment where data-driven decision-making and continuous learning are central to the organisation's ethos.
Dimension 4: Leadership Support
Leadership commitment to AI is crucial for successful transformation. The scorecard evaluates executive commitment, AI vision and sponsorship, risk tolerance, long-term commitment, and resource allocation.
Organisations with strong executive commitment to AI and clearly defined AI visions are more likely to lead in AI innovation. Leadership’s ability to support AI initiatives through strategic investment and appropriate risk-taking is pivotal in driving successful AI integration.
Dimension 5: AI Strategy and Planning
An effective AI strategy must align with overall business objectives. The evaluation criteria include strategic alignment, use case prioritisation, implementation roadmap, measurement framework, and portfolio management.
Organisations need a well-defined AI strategy that prioritises high-impact use cases, integrates AI initiatives into the broader business strategy, and tracks success through comprehensive metrics. A structured implementation roadmap and portfolio management framework ensure that AI projects are well-coordinated and aligned with business goals.
Dimension 6: Ethical and Social Considerations
Ethical considerations in AI are becoming increasingly important. This dimension assesses the organisation's approach to AI ethics, bias and fairness, transparency and explainability, privacy and data protection, and societal impact.
Organisations must ensure that AI systems are developed with fairness, transparency, and privacy in mind. A proactive approach to ethical AI, addressing bias, and assessing the societal impact of AI technologies is essential for long-term success.
Dimension 7: Talent and Skills Ecosystem
AI success depends heavily on the availability of skilled talent. This dimension evaluates AI expertise, technical skills, domain knowledge integration, talent acquisition and retention, and training and development.
Organisations should focus on developing AI expertise across technical and business functions, integrating domain knowledge into AI solutions, and creating robust talent acquisition and retention strategies. A strong focus on continuous learning and development will ensure that the organisation can adapt to evolving AI technologies.
Scoring and Interpretation
To calculate the readiness score, organisations rate themselves on each criterion, calculate dimension scores by averaging the criteria scores, and then calculate the overall AI readiness score. The overall score will place the organisation in one of the following stages:
- 1.0-2.0: Nascent Stage — Significant work needed
- 2.1-3.0: Emerging Stage — Foundation established but significant development required
- 3.1-4.0: Developing Stage — Moderate capabilities with targeted improvements needed
- 4.1-4.5: Advanced Stage — Strong capabilities with refinement opportunities
- 4.6-5.0: Leading Stage — Exceptional capabilities positioned for AI leadership
Dimension Analysis
The scorecard provides valuable insights beyond the overall score:
- Identify low-scoring dimensions to prioritise for improvement.
- Analyse patterns across dimensions to identify systemic issues.
- Recognise high-scoring dimensions to leverage organisational strengths.
- Compare scores across business units to identify best practices.
Action Planning
To address identified gaps, organisations should:
- Assess the impact of addressing each gap
- Estimate the effort and resources required
- Identify dependencies and establish appropriate timeframes
- Define specific improvement objectives, success metrics, and ownership
- Create milestone-based implementation plans with regular reviews
Case Studies: AI Readiness in Action
Case Study 1: UNESCO AI Readiness Assessment Methodology
UNESCO’s AI Readiness Assessment Methodology was employed by a ministry of education in a middle-income country to evaluate its readiness for AI integration in education. The assessment revealed several key gaps: a moderate policy framework (3.2), underdeveloped infrastructure in rural areas (2.4), strong capacity development through teacher training (3.8), but gaps in ethical frameworks (2.1) and equity (2.3).
To address these issues, the ministry focused on creating ethical guidelines for AI in education, improving infrastructure in underserved areas, and developing an AI curriculum. A cross-ministerial governance structure was also established to oversee the integration of AI across the education system. Over a two-year period, significant progress was made. The ethical framework improved from 2.1 to 3.9, infrastructure disparities between urban and rural areas were reduced, and the AI curriculum reached over 500 teachers.
The initiative successfully piloted AI-powered adaptive learning systems in 200 schools, improving student outcomes and increasing access to AI-driven education. This case demonstrates how systematic assessments and targeted improvements can lead to meaningful progress in AI adoption, balancing technology with social responsibility.
Case Study 2: Microsoft AI Business School Assessment
A multinational retail organisation used Microsoft’s AI Business School Assessment framework to evaluate its AI readiness. The assessment highlighted regional discrepancies in strategy alignment (2.3-4.1), cultural challenges around data-driven decision-making (2.8), and strong capabilities in e-commerce (4.2) but weaker AI adoption in logistics and marketing. The company also recognised the need to strengthen its responsible AI practices (3.0).
In response, the organisation developed a unified AI strategy that aligned with business objectives and launched a cultural transformation programme led by executive sponsors. A centre of excellence (CoE) was created to transfer AI knowledge across divisions, particularly focusing on underperforming areas. A comprehensive responsible AI governance framework was also established to address ethical concerns and ensure fairness in AI applications.
After 20 months, the company reported a 40% improvement in AI strategy alignment, a cultural readiness increase to 3.7, and the successful deployment of 15 AI use cases, including inventory optimisation and personalised marketing. These initiatives resulted in £142M in documented business value. This case underscores the importance of a structured transformation programme and responsible AI practices in achieving AI maturity across a large organisation.
Benchmarking and Industry Standards
Organisations can compare their scores against industry benchmarks. The current industry average AI readiness scores are:
- Financial Services: 3.7
- Healthcare: 3.2
- Manufacturing: 3.0
- Retail: 3.4
- Technology: 3.9
- Government: 2.8
- Education: 2.6
Continuous Improvement
AI readiness is an ongoing process. Organisations should reassess their AI readiness regularly (every 6-12 months), track progress, adjust priorities, and incorporate lessons learned into future planning. Staying informed of evolving best practices and standards is essential for continuous improvement.
The Path Forward
The AI Readiness Scorecard provides a structured approach for organisations to assess their state and plan for AI adoption. Organisations that succeed will be those that approach AI not merely as a technological upgrade, but as a strategic transformation across people, processes, technology, and culture. By addressing readiness across all dimensions, organisations can move beyond isolated AI projects and create sustainable value.
Share this
Dinis Guarda
Author
Dinis Guarda is an author, entrepreneur, founder CEO of ztudium, Businessabc, citiesabc.com and Wisdomia.ai. Dinis is an AI leader, researcher and creator who has been building proprietary solutions based on technologies like digital twins, 3D, spatial computing, AR/VR/MR. Dinis is also an author of multiple books, including "4IR AI Blockchain Fintech IoT Reinventing a Nation" and others. Dinis has been collaborating with the likes of UN / UNITAR, UNESCO, European Space Agency, IBM, Siemens, Mastercard, and governments like USAID, and Malaysia Government to mention a few. He has been a guest lecturer at business schools such as Copenhagen Business School. Dinis is ranked as one of the most influential people and thought leaders in Thinkers360 / Rise Global’s The Artificial Intelligence Power 100, Top 10 Thought leaders in AI, smart cities, metaverse, blockchain, fintech.
previous
Cybersecurity Funding Reaches $7.7 Billion in Q1 2025, Signalling Sector Rebound and Investor Confidence
next
What's the Difference Between College and University? A Clear Guide