business resources
Industrial AI: From Concepts To Industry Specifics
7 Jun 2024, 8:41 am GMT+1
Industrial AI is one the top performing technologies that industries are now adopting 360. With global corporations like Siemens, HubSpot, IBM, General Electric, and Bosch, amongst others, innovating in integrating the technology within its operations, the Industrial AI market is expected to reach $45-60 billion by 2029.
The case of Industrial AI is transversal to any industry or sector and companies such as Workflow automation platform, ServiceNow, is achieving case avoidance rates of nearly 20% with their AI-powered Now Assist. Other companies such as Palo Alto Networks have reduced the cost of processing expenses with AI. Giant marketing and data, HubSpot, has scaled customer support with AI. And companies such as Swedish fintech Klarna recently announced over $40 million in run-rate savings by building AI into their customer support.
In the next 5 years (2024-2029), the industrial AI market is expected to grow at a compound annual growth rate (CAGR) of 25-30%, reaching $45-60 billion by 2029. The adoption of industrial AI is expected to accelerate across various sectors, with manufacturing, energy, and transportation being the leading adopters. The ecosystem will likely consist of a mix of established technology providers, industrial giants, startups, and research institutions collaborating to develop and deploy AI solutions.
“We are entering an Industrial AI revolution world where”, as NVIDIA CEO Jensen Huang says, “every pixel will be generated by AI!”
Industrial AI: From concepts to industry specifics
Industrial AI, also known as Industrial Artificial Intelligence, refers to the application of artificial intelligence technologies within the context of industrial processes, manufacturing, and supply chain management. It encompasses a wide range of AI techniques, including machine learning, deep learning, computer vision, natural language processing, and robotics, among others, to optimise and enhance various aspects of industrial operations.
Unlike general artificial intelligence which is a frontier research discipline to build computerised systems that perform tasks requiring advanced human intelligence, Industrial AI is more concerned with the application of such technologies to address industrial pain-points for customer value creation, productivity improvement, cost reduction, site optimisation, predictive analysis and insight discovery.
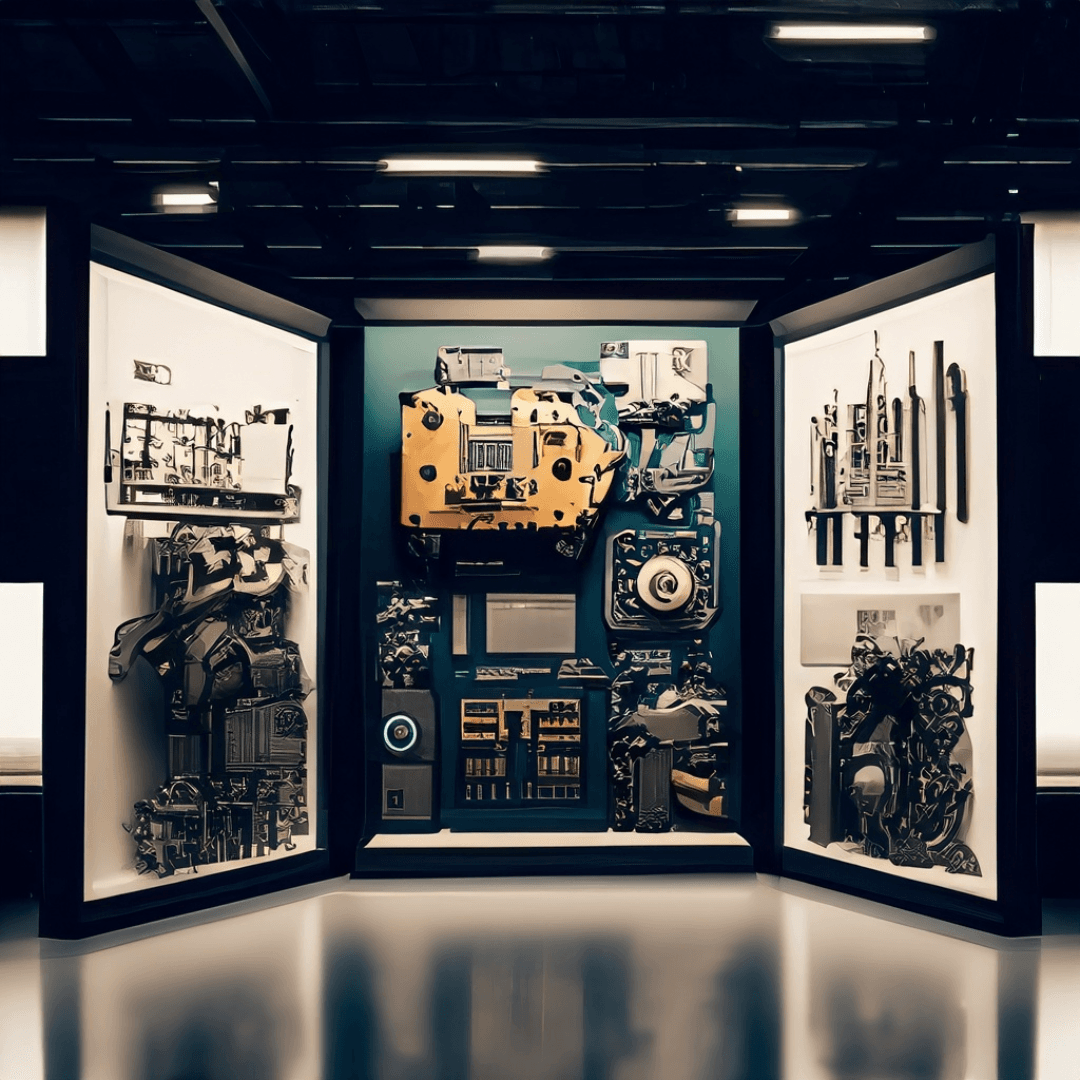
Some key areas where Industrial AI finds application include:
Predictive Maintenance: AI algorithms can analyse historical data, machine performance metrics, and sensor readings to predict potential equipment failures before they occur. This enables proactive maintenance, reducing downtime and minimising repair costs.
Quality Control: AI-powered computer vision systems can inspect products and detect defects or anomalies in real-time, ensuring consistent quality throughout the manufacturing process. This helps minimise waste, improve customer satisfaction, and maintain high standards of production.
Process Optimisation: AI can analyse production data, identify bottlenecks, and suggest improvements to streamline manufacturing processes. By optimising resource allocation, scheduling, and inventory management, AI can enhance overall operational efficiency and reduce costs.
Supply Chain Management: AI algorithms can analyse vast amounts of data from various sources, such as market trends, customer demand, and supplier performance, to optimise supply chain operations. This includes demand forecasting, inventory optimization, and logistics planning, leading to improved responsiveness and agility in the supply chain.
Autonomous Systems: Industrial AI can enable the development of autonomous robots and vehicles that can perform tasks without human intervention. These systems can operate in hazardous environments, handle repetitive tasks, and collaborate with human workers to enhance productivity and safety.
The concept of Industrial AI is closely tied to the broader framework of Industry 4.0, which represents the fourth industrial revolution. Industry 4.0 envisions the integration of advanced technologies, including AI, Internet of Things (IoT), cloud computing, and cyber-physical systems, to create smart factories and intelligent manufacturing ecosystems.
Implementing Industrial AI requires a comprehensive approach that involves data collection, preprocessing, model development, deployment, and continuous monitoring. It also necessitates a strong focus on data security, privacy, and ethical considerations, as the use of AI in industrial settings can have significant implications for workers, businesses, and society as a whole.
Industrial AI for businesses: A case study of Siemens
AI and especially Industrial AI, at large, are positioned to change the overall life cycle of businesses and sectors and optimise and manage cost structure and increase productivity in some of the most crucial areas in our society. Industrial AI has the potential to lead to better education, healthier populations and more productive people by abstracting away mundane work and allowing us to focus our attention on more important issues and better tools for the future. It can free up more people to tackle more problems to create a better society.
The use cases are wide-ranging and far-reaching, as immediately evident from the three largest companies on the list in terms of valuation. Model maker OpenAI ($86 billion) counts customers from Morgan Stanley to the government of Iceland, while its rival Anthropic ($18.4 billion, as Forbes reported) is used by Bridgewater and the Boston Consulting Group. Databricks ($43 billion) sells its data analytics and AI deployment software to Shell and the United States Postal Service. For the startups on AI 50, the technology has evolved from capturing customers’ imaginations to capturing billions of dollars in collective revenue.
Industry giants like Siemens are at the forefront of advancing industrial AI applications, with a notable initiative, Siemens MindSphere. It is an open, cloud-based IoT operating system that enables the collection, analysis, and visualisation of industrial data using AI and machine learning techniques. Through MindSphere, Siemens facilitates more informed decision-making and enhanced operational efficiencies for its clients.
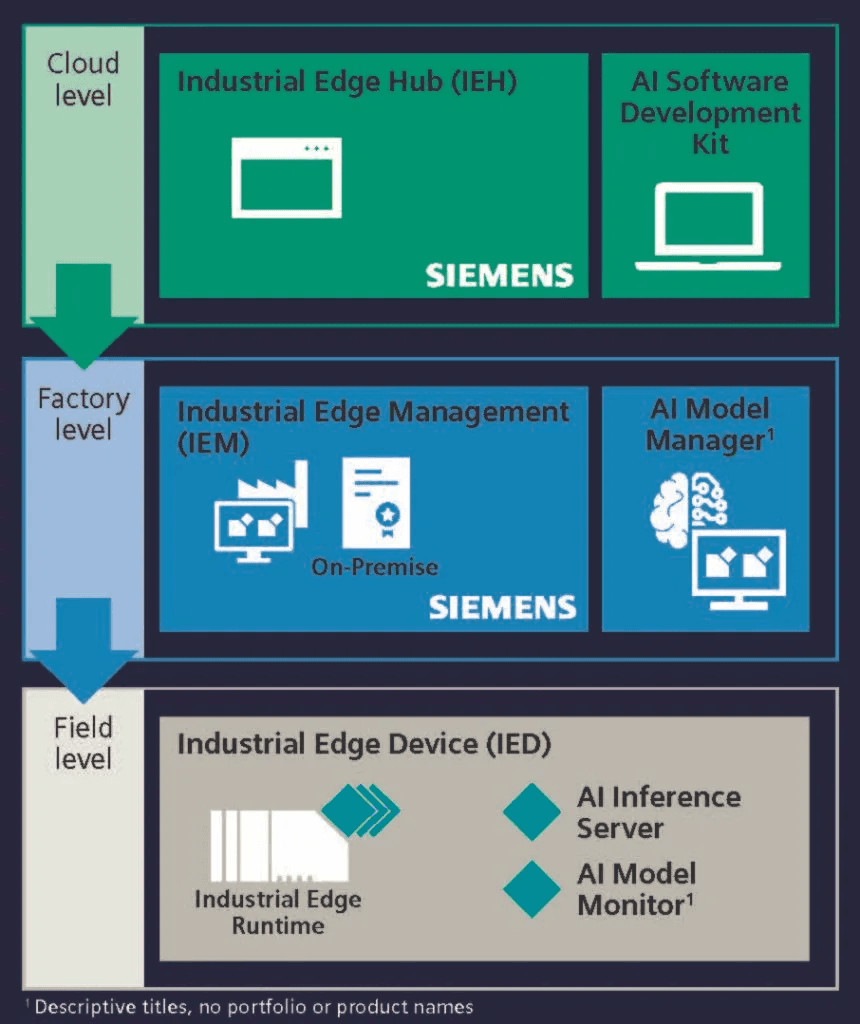
Siemens also develops AI-powered predictive maintenance solutions. These solutions analyse sensor data to predict equipment failures and optimise maintenance schedules. By anticipating potential issues before they occur, Siemens helps companies reduce downtime and maintenance costs while extending the lifespan of their equipment.
Another significant area of focus for Siemens is AI-driven autonomous systems for industrial applications. This includes the development of self-driving vehicles for material handling and autonomous robots for assembly tasks. These autonomous systems aim to increase productivity and safety in industrial environments by reducing the need for human intervention in repetitive and hazardous tasks.
Siemens has also used AI to create digital twins, which are digital replicas of physical assets, processes, and systems. These digital twins enable real-time monitoring, simulation, and optimisation of industrial operations. By providing a virtual representation of physical entities, Siemens helps businesses improve their operational efficiency and make more accurate predictions about future performance.
Global corporations investing in Industrial AI applications
Several global companies are investing heavily in industrial AI, driving innovations and offering cutting-edge products and solutions.
Siemens, based in Germany, has invested billions in R&D for digital industries, including industrial AI. The company's innovations include Siemens MindSphere, an IoT platform, AI-powered predictive maintenance, and autonomous systems. Among its products are MindSphere, Siemens Industrial Edge, and Siemens NX, a CAD/CAM software with AI capabilities.
General Electric (GE) in the USA has also made significant investments in AI research and its Predix platform for industrial IoT. GE's innovations feature the Predix industrial IoT platform and AI-powered asset performance management. Its product offerings include Predix, GE Digital Twin, and the GE Brilliant Manufacturing Suite.
IBM, another prominent player from the USA, has invested extensively in AI research and its Watson platform for industrial applications. IBM's innovations comprise IBM Watson for Industry, an AI platform, predictive maintenance, and quality control. Its key products are IBM Watson for Industry and IBM Maximo, an asset management software with AI capabilities.
Bosch, headquartered in Germany, has focused on AI research and its IoT platform, Bosch IoT Suite. The company's innovations include the Bosch IoT Suite industrial IoT platform, AI-powered predictive maintenance, and autonomous systems. Its product lineup features the Bosch IoT Suite and Bosch Rexroth, offering automation solutions with AI capabilities.
Hitachi from Japan has invested in AI research and its Lumada platform for industrial IoT. Hitachi's innovations encompass the Lumada industrial IoT platform, AI-powered predictive maintenance, and optimisation solutions. Notable products from Hitachi include Lumada, Hitachi Industrial AI, and Hitachi Vantara, which provides data management solutions with AI capabilities.
Schneider Electric in France has invested in AI research and its EcoStruxure platform. Schneider Electric's innovations include the EcoStruxure IoT-enabled architecture, AI-powered predictive maintenance, and energy optimisation. Its key products are EcoStruxure and Schneider Electric PlantStruxure, a process automation system with AI capabilities.
Rockwell Automation in the USA has focused on AI research and its FactoryTalk platform. The company's innovations feature the FactoryTalk industrial IoT platform, AI-powered predictive maintenance, and optimisation solutions. Among its products are FactoryTalk and Rockwell Automation PlantPAx, a distributed control system with AI capabilities.
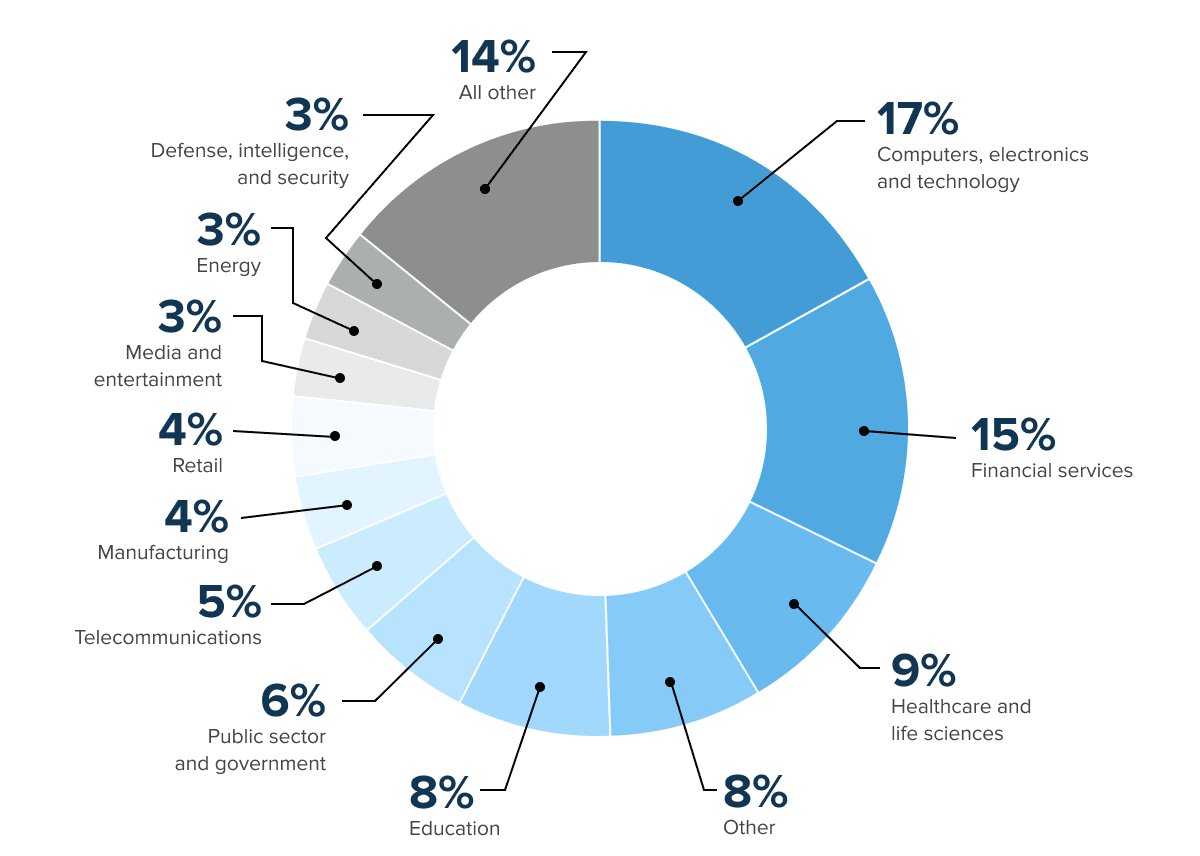
The systemic risks and impacts of Industrial AI
As industrial AI scales across major industries and sectors, it brings a range of potential systemic risks that must be carefully considered and addressed.
One significant risk is job displacement and economic disruption. The increasing automation of tasks and processes through industrial AI could lead to significant job losses, particularly in labour-intensive sectors such as manufacturing, transportation, and retail. This could result in economic instability, income inequality, and social unrest if not managed properly.
Algorithmic bias and discrimination also pose serious concerns. AI systems are only as unbiased as the data they are trained on and the algorithms they employ. If industrial AI systems are trained on biased data or use algorithms that perpetuate discriminatory practices, they could exacerbate existing inequalities and lead to unfair treatment of certain groups.
Safety and reliability concerns are another critical issue. As industrial AI systems become more complex and autonomous, there is a risk of unexpected behaviours or failures that could compromise safety and reliability. The lack of transparency and explainability in some AI systems could make it difficult to identify and rectify problems, potentially leading to accidents or disasters.
Cybersecurity vulnerabilities present further challenges. The increasing connectivity and integration of industrial AI systems with other technologies and networks could create new cybersecurity risks. Cyber-attacks on industrial AI systems could have cascading effects across supply chains, critical infrastructure, and essential services, causing widespread disruption and damage.
Data privacy and ownership are also at risk. The deployment of industrial AI relies on the collection and analysis of vast amounts of data, including sensitive information about individuals, organisations, and assets. The lack of clear data privacy regulations and ownership frameworks could lead to the misuse or exploitation of this data, compromising individual rights and corporate intellectual property.
The concentration of power and monopolistic practices is another concern. The development and deployment of industrial AI solutions are largely driven by a few major technology companies and industrial giants. This concentration of power could lead to monopolistic practices, limiting competition and innovation, and potentially exacerbating existing power imbalances.
Geopolitical tensions and the AI arms race are also potential risks. The race to develop and control advanced industrial AI technologies could fuel geopolitical tensions and an AI arms race between nations. The use of industrial AI for military purposes or the hoarding of AI talent and resources by certain countries could create new sources of conflict and instability.
Environmental impact and resource consumption must also be considered. The training and deployment of large-scale industrial AI systems require significant computational resources and energy consumption. If not managed sustainably, the widespread adoption of industrial AI could contribute to increased carbon emissions and environmental degradation.
Ethical and moral dilemmas are inherent in the use of industrial AI in decision-making processes. These dilemmas could arise in situations where AI systems are tasked with making choices that have significant consequences for individuals, communities, or the environment.
Lack of governance and accountability is also a pressing issue. The rapid pace of industrial AI development and deployment could outpace the ability of governments and regulators to establish effective governance frameworks and accountability mechanisms. This could create a regulatory vacuum, allowing for the unchecked development and use of industrial AI systems.
To mitigate these systemic risks, it is crucial for policymakers, industry leaders, and other stakeholders to engage in proactive dialogue and collaboration to develop responsible and inclusive approaches to industrial AI. This includes investing in research and development to address technical challenges, establishing clear ethical guidelines and standards, and creating governance frameworks that ensure accountability and transparency.
By proactively addressing these systemic risks, we can harness the potential of industrial AI to drive innovation, efficiency, and progress while minimising negative consequences and ensuring that the benefits of this transformative technology are shared equitably across society.
Scaling Industrial AI
To ensure that industrial players can effectively scale the best of industrial AI and avoid getting lost in the rapid pace of development, they should start by defining clear objectives and identifying specific use cases where AI can deliver the most value. This helps in prioritising investments and ensuring that AI initiatives are aligned with business goals.
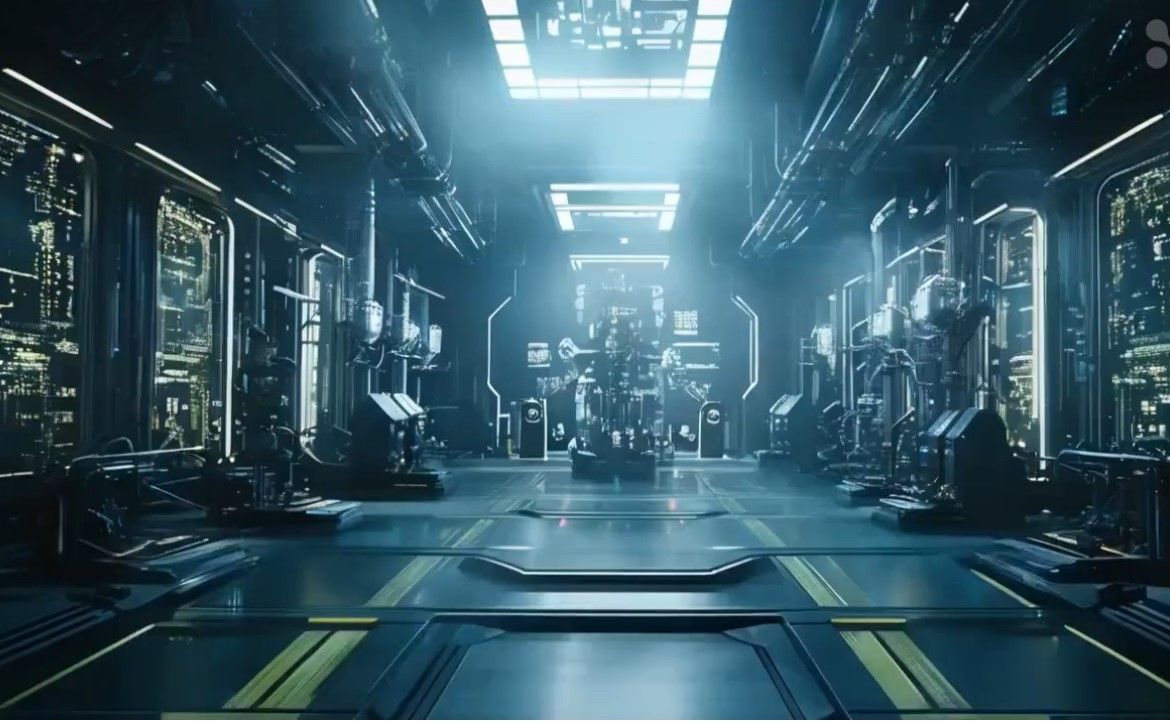
Developing a robust data strategy is crucial, as industrial AI relies heavily on high-quality, relevant data. Companies should invest in data collection, storage, governance, and security to ensure that AI systems have access to the right data to generate meaningful insights and make accurate predictions.
Fostering a culture of innovation and collaboration is essential for scaling industrial AI. Industrial players should create an environment that supports cross-functional teams, encourages knowledge sharing, and rewards innovation. This culture should encourage experimentation, learning, and collaboration across different functions and levels of the organisation.
Investing in talent and skills development is another key strategy. The successful deployment of industrial AI requires a mix of domain expertise, technical skills, and business acumen. Industrial players should invest in training and upskilling their workforce to build the necessary capabilities in-house, and consider partnering with academic institutions and technology providers to access specialised talent and expertise.
Establishing strong governance and ethical frameworks is vital as industrial AI systems become more autonomous and influential. This includes developing guidelines for data privacy, algorithmic accountability, and human oversight, as well as regularly auditing AI systems for fairness and bias to ensure responsible and transparent deployment.
Adopting an agile and iterative approach to implementation is necessary due to the rapid pace of industrial AI development. Industrial players should start with small-scale pilots and proof-of-concepts, learn from the results, and iterate based on feedback. This allows for faster learning, reduces risk, and enables continuous improvement.
Collaboration with the broader ecosystem is also important for scaling industrial AI. Industrial players should actively seek out opportunities for collaboration and co-innovation with technology providers, domain experts, and industry partners. Leveraging the strengths and expertise of different stakeholders can accelerate development and adoption.
Monitoring and measuring performance is crucial to ensure that industrial AI initiatives deliver the desired outcomes. This involves establishing clear performance metrics and regularly tracking key performance indicators related to efficiency, quality, safety, and other relevant metrics. Regularly assessing the impact of AI on business outcomes is also essential.
Communicating and engaging with stakeholders is necessary to gain buy-in and support for scaling industrial AI. Industrial players should proactively communicate the benefits and potential impacts of AI, engage in dialogue to address concerns and incorporate feedback, and build trust through transparency and accountability.
Planning for long-term sustainability is an essential step for the adoption of industrial AI. Industrial players should consider not just the immediate benefits but also the potential risks and implications for the future. This includes planning for the ethical and social implications of AI, investing in research and development to address long-term challenges, and building resilience and adaptability into AI systems and processes.
Our future with Industrial AI
Thousands of companies are now integrating AI into their workflows, industry sectors and are scaling their businesses and operating technologies to see increased growth and decrease costs. AI companies are enabling these rapid improvements.
This is however just the beginning, and tomorrow, we expect to see entire industries and sectors integrate Industry AI and the full spectrum that comes with it. From heavy industry, to data and analytics UX and UI reimagined around the capabilities of AI in any department or systems around.
Share this
Dinis Guarda
Author
Dinis Guarda is an author, entrepreneur, founder CEO of ztudium, Businessabc, citiesabc.com and Wisdomia.ai. Dinis is an AI leader, researcher and creator who has been building proprietary solutions based on technologies like digital twins, 3D, spatial computing, AR/VR/MR. Dinis is also an author of multiple books, including "4IR AI Blockchain Fintech IoT Reinventing a Nation" and others. Dinis has been collaborating with the likes of UN / UNITAR, UNESCO, European Space Agency, IBM, Siemens, Mastercard, and governments like USAID, and Malaysia Government to mention a few. He has been a guest lecturer at business schools such as Copenhagen Business School. Dinis is ranked as one of the most influential people and thought leaders in Thinkers360 / Rise Global’s The Artificial Intelligence Power 100, Top 10 Thought leaders in AI, smart cities, metaverse, blockchain, fintech.
previous
Protect Your Blockchain Innovations with Expert Auditing Services
next
How To Manage Payroll Taxes For Contractors